Introduction:
In the evolving world of technology, Large Language Models (LLMs) like ChatGPT have been making headlines for their impressive capabilities in understanding and generating human-like text. But beyond language lies a more focused application for designers and developers: Large Language Design Models (LLDMs). These advanced models are poised to transform how we approach user interface (UI) and user experience (UX) design by integrating generative AI with design principles. As a Principal UI Architect, let’s delve into this emerging field, its maturity as a product, and its pros and cons.
What Are LLDMs?
LLDMs are AI models specialized for assisting with design-related tasks. Unlike general-purpose LLMs, LLDMs are fine-tuned on vast datasets of UI frameworks, design patterns, usability studies, and even aesthetic principles. Think of them as AI-powered co-designers that can generate wireframes, suggest layout optimizations, and even predict user interaction issues based on heuristics.
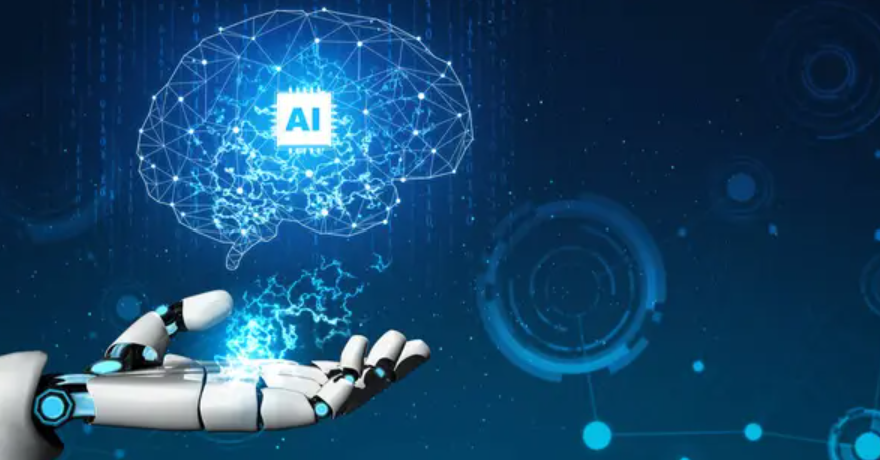
How LLDMs Will Mature as a Product
LLDM technology is currently in its nascent stages, often integrated into broader design tools like Figma or Adobe XD. However, here’s how it’s likely to evolve:
- Contextual Design Generation: Models will become adept at understanding specific design contexts, such as industry standards, accessibility requirements, and branding guidelines, to produce tailored recommendations.
- Collaborative Workflows: LLDMs will integrate seamlessly with team workflows, enabling real-time collaboration by suggesting improvements during design sprints.
- Explainable AI in Design: Future LLDMs will provide detailed explanations for their suggestions, making it easier for designers to trust and refine AI-generated outputs.
- Feedback Loops: The ability to learn from user feedback and improve iteratively will enhance their precision and relevance over time.
- Cross-Disciplinary Applications: Beyond UI/UX, LLDMs could assist with product strategy by simulating user behaviors, improving onboarding flows, and aligning designs with business goals.
Advantages of LLDMs
- Speed and Efficiency: LLDMs can generate design prototypes in minutes, dramatically reducing the initial ideation phase.
- Data-Driven Insights: They analyze vast datasets to make recommendations, helping eliminate guesswork in design.
- Scalability: Whether you’re designing for mobile, web, or IoT, LLDMs can adapt designs for multiple platforms effortlessly.
- Accessibility Optimization: LLDMs are trained to consider accessibility standards like WCAG, making inclusive design more achievable.
- Cost Reduction: By streamlining repetitive tasks, LLDMs free up human designers for more strategic, creative work.
Disadvantages and Drawbacks
- Creativity Constraints: LLDMs work within the boundaries of their training data, which may limit innovation or lead to designs that feel formulaic.
- Learning Curve: Integrating LLDMs into workflows may require training and adjustments, especially for teams new to AI tools.
- Dependence on Data Quality: The quality of LLDM outputs depends heavily on the datasets they’re trained on. Biases or gaps in the data could lead to suboptimal designs.
- Ethical Concerns: Over-reliance on AI for design decisions could raise questions about authorship and accountability.
- Job Displacement Fears: While LLDMs are meant to augment human creativity, there’s a risk of designers feeling threatened by automation.
The Path Forward
As LLDMs continue to mature, the role of designers will shift. Instead of solely crafting interfaces, designers will act as orchestrators—guiding AI tools to align outputs with user needs and business objectives. The future lies in collaboration, where LLDMs enhance creativity rather than replace it.
For organizations, adopting LLDMs means staying ahead in a competitive digital landscape. By leveraging these tools, teams can iterate faster, deliver user-centered designs, and adapt to ever-changing market demands.
Conclusion:
Large Language Design Models represent the next wave of innovation in the UI/UX space. While challenges exist, the potential to revolutionize design workflows is undeniable. As we embrace these tools, the emphasis must remain on creating meaningful, human-centered experiences—because at the end of the day, even the smartest LLDM is only as good as the vision guiding it.
Are you ready to design with the future in mind?